Artificial intelligence (AI) has rapidly advanced in recent years, enabling breakthroughs in various fields. In the realm of AI generated art, transfer learning techniques have emerged as a powerful tool to enhance creativity and diversity. Transfer learning can artificially introduce sample diversity through data augmentation techniques, applying random and realistic transformations to the training images to improve model performance and prevent overfitting. This article delves into the fascinating world of transfer learning and investigates its application in AI art, unlocking new possibilities for artistic expression.
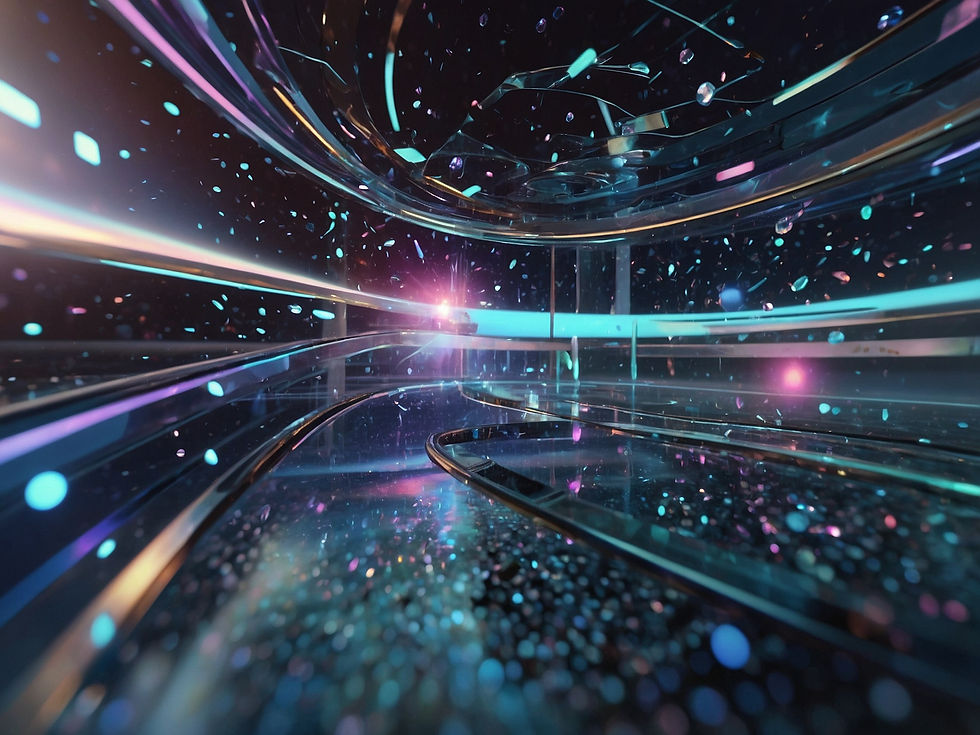
What is Transfer Learning in a Pre Trained Model?
Transfer learning (TL) is a machine learning technique that leverages the knowledge gained from one task or dataset to enhance a model’s performance on a related task or dataset. This approach is particularly popular in deep learning because it allows deep neural networks to be trained with less data than starting from scratch. Essentially, TL makes it possible to build efficient models without needing enormous amounts of new data.
The process of TL involves fine-tuning a pre-trained model to tackle a new, but related, task. For example, a neural network initially trained to identify handbags can be adapted to recognize wallets or sunglasses by retraining only its final layers, often excluding the classification layer to leverage the model's ability to extract general features. This technique improves learning in the target domain by leveraging existing knowledge from the source domain, enabling quicker parameter tuning, and enhancing generalization capabilities. In essence, TL is about borrowing expertise from one domain to achieve faster and more effective learning in another.
Definition and Explanation
Transfer learning is a groundbreaking machine learning technique where a pre-trained model serves as the starting point for a new task. Instead of building a model from scratch, transfer learning leverages the knowledge and features learned from the initial task to improve performance on the new one. By utilizing a pre-trained model, this approach saves significant time and resources, as the model has already acquired general features that are applicable to a wide range of tasks. This method not only accelerates the learning process but also enhances the model’s ability to adapt to new challenges, making it a powerful tool in the realm of AI art.
Understanding Transfer Learning
Transfer learning is a technique that leverages knowledge gained from one task to improve performance on another related task. By utilizing a pre-trained network, AI models can learn from pre-existing knowledge and adapt it to new contexts. In the realm of AI art, transfer learning allows models to benefit from existing artistic styles, patterns, and features, fostering creativity and diversity in the generated artwork.
Enhancing Creativity in AI Art
Transfer learning enables AI models to learn from a vast collection of existing artwork, including renowned masterpieces, contemporary pieces, or even personal artistic styles. The input data used in training these models plays a crucial role in shaping their ability to learn and adapt. By extracting and transferring the knowledge captured in these artworks, the models gain a broader understanding of artistic concepts, such as composition, color usage, or texture. This expanded knowledge base enhances the creative potential of AI systems, enabling them to generate unique and visually stunning art pieces.
Artificially Introduce Sample Diversity through Style Transfer
Style transfer is an exciting application of transfer learning in AI art. The training process involves compiling and training models, monitoring performance through techniques like early stopping, and adjusting weights and hyperparameters to improve the model's performance and prevent overfitting. It allows artists and AI systems to merge different artistic styles to create visually captivating and eclectic artworks. By combining the style of one artwork with the content of another, AI models can produce novel compositions that fuse diverse artistic influences. This process not only promotes artistic diversity but also encourages experimentation and the creation of hybrid art forms that push the boundaries of traditional styles.
Transcending Artistic Domains through Fine Tuning
Transfer learning techniques in AI art are not limited to visual arts alone. They can be applied across different artistic domains, including music, literature, or even dance. Frozen layers play a crucial role in preserving learned features when applying transfer learning across these domains. For instance, a model trained on a large corpus of music compositions can learn intricate musical patterns and harmonies, enabling it to generate original melodies or compose pieces inspired by specific musical genres. This interdisciplinary approach fosters cross-pollination of ideas, opening up new avenues for artistic exploration.
Collaboration and Co-Creation
Transfer learning in AI art facilitates collaboration and co-creation between human artists and intelligent systems. Artists can harness the power of pre-trained models, leveraging their artistic knowledge and merging it with their own creative instincts. Fine-tuning the entire model is crucial to enhance performance in collaborative AI art projects, ensuring that the synergy between human creativity and machine intelligence results in innovative and thought-provoking artworks, pushing the boundaries of artistic expression.
Transfer learning has revolutionized the field of AI-generated art, providing a pathway to enhance creativity and diversity. By leveraging pre-existing knowledge and artistic styles, AI systems can generate captivating and unique artwork that expands the horizons of artistic expression. Through the collaborative interplay between artists and AI, transfer learning techniques pave the way for a future where human imagination and machine intelligence converge, reshaping the landscape of art creation.
Benefits of Transfer Learning
Transfer learning offers a multitude of benefits that make it an invaluable technique in the field of AI art:
Enhanced Efficiency: One of the most significant advantages of transfer learning is its ability to drastically reduce the time and resources required to train a machine learning model. By starting with a pre-trained model, the learning process is accelerated, allowing the model to adapt to new tasks more swiftly. This efficiency is particularly beneficial when dealing with complex artistic styles and large datasets.
Improved Performance: Transfer learning can significantly boost the performance of a machine learning model. By leveraging the knowledge and features learned from the initial task, the model can achieve better accuracy and robustness. This is especially important in AI art, where the ability to capture intricate details and nuances can make a substantial difference in the quality of the generated artwork.
Increased Accessibility: Transfer learning democratizes the field of machine learning by making it more accessible to organizations with limited resources. By using pre-trained models, even those without extensive computational power or large amounts of training data can develop sophisticated machine learning models. This opens up new opportunities for smaller organizations and individual artists to explore AI-generated art.
Enhanced Efficiency and Accessibility
Transfer learning enhances efficiency and accessibility in several impactful ways:
Reduced Training Time: One of the primary benefits of transfer learning is the reduction in training time. By starting with a pre-trained model, the learning process is significantly accelerated. This allows the model to quickly adapt to new tasks, making it possible to generate high-quality AI art in a shorter timeframe.
Improved Resource Utilization: Transfer learning optimizes resource utilization by minimizing the need for large amounts of training data and computational resources. This is particularly advantageous for organizations with limited resources, as it enables them to develop and deploy machine learning models without the need for extensive infrastructure.
Increased Model Robustness: By leveraging the knowledge and features learned from the initial task, transfer learning enhances the robustness of machine learning models. This results in better accuracy and more reliable performance, which is crucial for creating high-quality AI-generated art that can capture the intricacies of various artistic styles.
Popular Pre-Trained Models
Several popular pre-trained models are widely used in transfer learning, each offering unique advantages for different tasks:
Convolutional Neural Networks (CNNs): CNNs are a type of neural network particularly well-suited for image classification tasks. They can be pre-trained on large datasets like ImageNet and then fine-tuned for specific tasks, such as object detection and image segmentation. CNNs excel in feature extraction, making them ideal for tasks that require detailed analysis of visual data.
Recurrent Neural Networks (RNNs): RNNs are commonly used for natural language processing tasks. They can be pre-trained on extensive datasets such as Wikipedia and fine-tuned for specific applications, including text generation and sentiment analysis. RNNs are adept at handling sequential data, making them valuable for tasks that involve understanding and generating human language.
Transformers: Transformers have revolutionized natural language processing with their ability to handle long-range dependencies in text. Pre-trained on vast datasets like Wikipedia, transformers can be fine-tuned for a variety of tasks, including translation, summarization, and question answering. Their versatility and performance make them a popular choice for many NLP applications.
Convolutional Neural Networks (CNNs)
Convolutional Neural Networks (CNNs) are a powerful type of neural network commonly used for image classification tasks. Pre-trained on large datasets such as ImageNet, CNNs can be fine-tuned for specific tasks, significantly enhancing their performance. CNNs are particularly useful for:
Image Classification: CNNs excel in image classification tasks, such as object detection and image segmentation. By learning to recognize patterns and features in images, they can accurately classify and identify various objects within a visual scene.
Feature Extraction: CNNs are highly effective at feature extraction, making them ideal for tasks that require detailed analysis of visual data. They can identify and extract important features from images, such as edges, textures, and shapes, which can then be used for further processing or analysis.
Image Generation: CNNs can also be used for image generation tasks, such as image synthesis and manipulation. By learning the underlying patterns and structures of images, CNNs can generate new images that are visually coherent and realistic.
By leveraging pre-trained CNNs, artists and developers can reduce the need for large amounts of training data and computational resources, making it easier to create high-quality AI-generated art.
Further Reading:
- Beginners Guide to Artificial Intelligence Artwork
Artificial Intelligence Artwork a comprehensive guide exploring the nuances of AI art, the technologies that make it possible and the various forms it takes.
- Artificial Intelligence Art Generator: Everything You Need to Know
The Artificial Intelligence Art Generator, democratizing art and allowing users to generate stunning visuals simply by inputting textual prompts in seconds.
- Leonardo AI Tutorial: Ultimate Guide to Creating AI Art Like a Pro
A comprehensive Leonardo AI tutorial designed to swiftly acquaint you with essential information and give guidance on creating amazing AI generated art with this free AI image generator.
- Guide to Mastering AI Art Prompts: AI Prompt Engineering Made Easy
Learn how to write effective AI art prompts with this easy to understand guide. Discover tips to unlock the full potential of your AI generated creations.
- Monetizing Creativity: Can You Sell AI Generated Art Successfully?
Can You Sell AI Generated Art? A guide that cuts through the legalese and unveils how to turn pixels into profit, clearly outlining the path to commercial success!
- The Ethics of AI Art: Addressing The AI Generated Elephant in The Room
The debate over AI art ethics rages on, so we're addressing the artificial elephant in the room. Discussing the complexities behind this controversial new art form.
Note: Most of the images used in this article were created using an AI art generator. So get out there and join the millions of other artists.
If you'd like to know more you can head over to AIArtKingdom.com for a curated collection of today's most popular, most liked AI artwork from across the internet. Plus explore an extensive array of AI tools, complemented by comprehensive guides and reviews, on our AI blog.